A Statistical Book Review for "Of Boys and Men" by Richard Reeves
Reeves' book makes a number of questionable statistical claims so I’ve reached out to him and suggested we do a predictive modeling competition to forecast future college enrollment rates.
One of the main focuses of Richard Reeves’ book, Of Boys and Men, is the educational underachievement of boys and the growing gap in college enrollment. The current gender ratio for college enrollment is approximately 70 men for every 100 women. To put that in perspective, after the First World War, the United Kingdom had 67 surviving men for every 100 women in the 20 - 29 age group. After the Second World War, Soviet Russia had 72 surviving men for every 100 women in the same age group.
Reeves attributes this massive disparity to boys not learning as well as girls in school, showing that boys are behind in reading and GPA scores, and he emphasizes that these are widening disparities. Reeves writes, “A denial of the large gender gap in education in favor of girls, especially in advanced economies, simply does not survive contact with the data.”
I decided to make contact.
Reeves is correct that there are gaps in reading, math, and GPA scores. But what I think is most notable is how incredibly persistent those gaps have been for as far back as we’ve measured them. Reeves incorrectly alleges that these gaps are widening. Reeves has missed the most fascinating part about the phenomenon: that the gendered learning gaps in grade school have remained incredibly consistent for decades, while our college campuses simultaneously transitioned to a gender ratio you’d expect to see if we just fought a world war on home soil using Soviet-era tactics.
I’ve reached out to Richard Reeves and suggested that we have a competition where we each try to forecast future enrollment gender ratios. If Reeves is confident about the impact of these learning gaps then he can use them to predict future enrollment rates. I have some ideas of my own.
Reeves says his goal is to “win hearts and minds” for the cause of helping boys and men in education. If Reeves is serious about wanting to change enrollment then he needs to understand the process well enough to predict it. Reeves is suggesting that a changing output variable (college enrollment gender gap) is somehow a function of a constant input variable (learning gender gaps). Forecasting future enrollment will emphasize that factors other than academic performance have driven the decline.
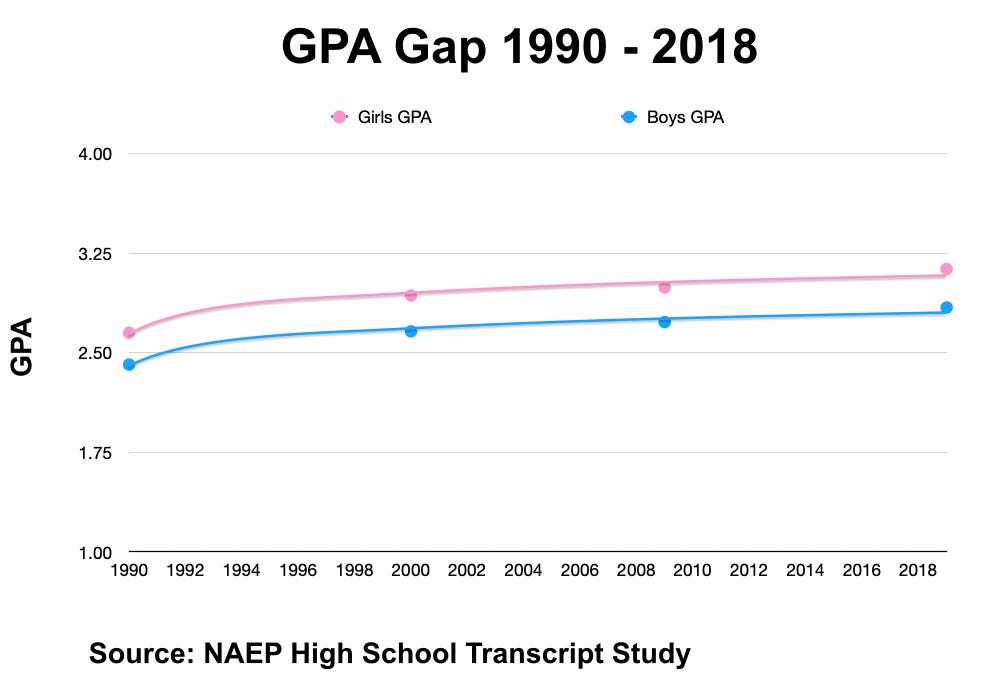
Reeves distorts the size of the gender gaps and continually portrays these learning gaps as widening, but provides no evidence to support that:
1) Reeves writes, “the [GPA] gap has widened in recent decades. The most common high school grade for girls is now an A; for boys, it is a B” (page 19). This is a misleading way of saying that they both grew proportionately and girls crossed a threshold where the most common grade is now an A.
One data visualization trick is to change the scale to make a small change look big or a big change look small. To avoid that I’ll put the min/max for each of the following charts by +/- 2 standard deviations.
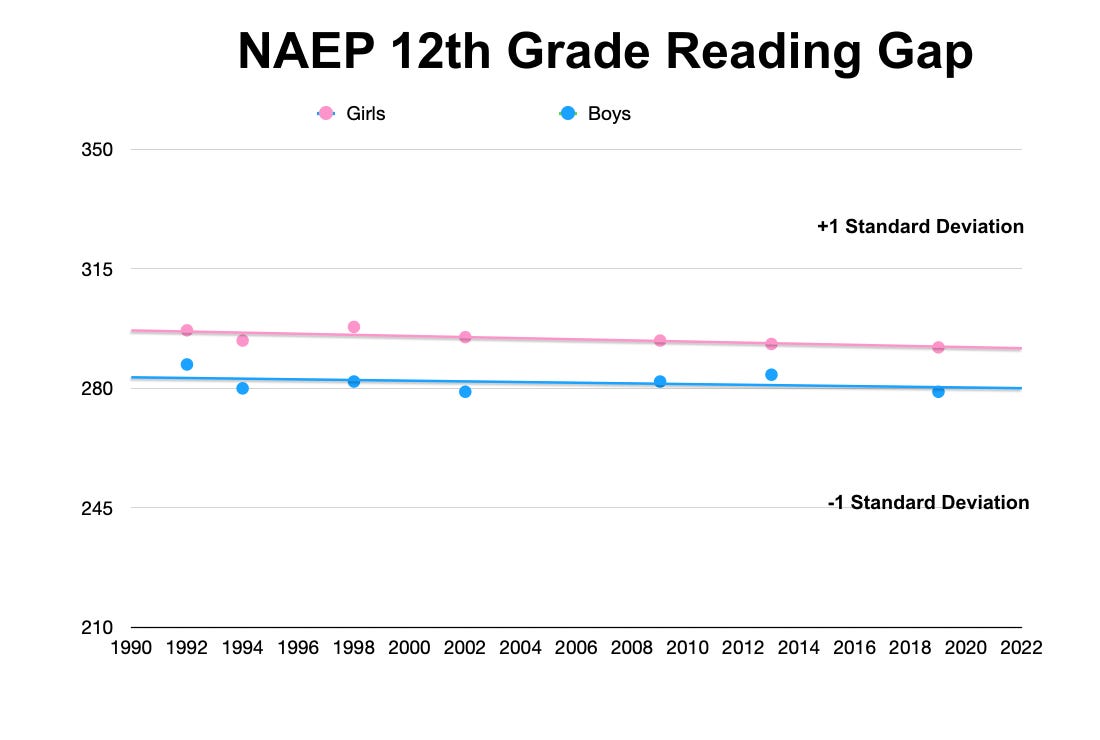
2) Reeves is correct that there are gaps, but does not put them into perspective. For example, he says that girls are about 13 points ahead in reading on the 12th grade NAEP reading test and boys are 3 points ahead on math. Okay, but how big of a difference is 13 points? This is roughly 1/3 of a standard deviation. To put this in perspective, the standard deviation for height is 2.3 inches. So one third of that is 0.8 inches. Imagine you walk into a room with a hundred people wearing green shirts and a hundred people wearing blue shirts, and I tell you that on average one group is 0.8 inches taller than the other. Just by looking around would you be able to readily figure out which group is taller? I probably couldn’t. Not giving this gap any sort of scale is a tried and true way of manipulating statistics to fit a narrative.
3) Reeves describes a common problem in statistical analysis, called the gap instinct (which is when you forget that the variance within one distribution is larger than the variance between two distributions) and then he makes this error himself. He cites a study that boys are two-thirds of a grade behind the girls in reading (page 18). The variance in NAEP within one grade level is much larger than the difference between two grades. Saying that they are 2/3 of a grade behind is equivalent to saying that the boys are 1/3 of a standard deviation behind. This is using the gap instinct to say the same thing but make it sound like a bigger difference than it actually is.
Reeves writes in a substack piece, “In the NAEP, there’s now no real gender gap on math and science (where girls have caught up to boys)”. He’s correct that there’s no real gap. But when he says now that implies this is a new situation. And to prove how much the girls have caught up at math and science, he then shows the historical scores on the reading section.
Let’s instead look at the math scores.
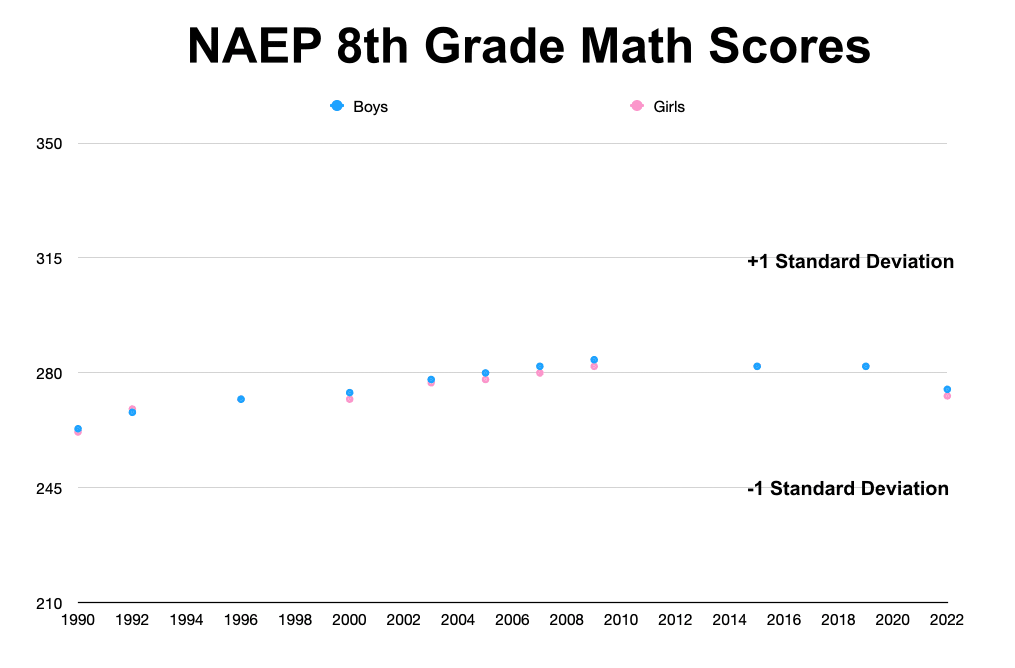
4) “There’s now no gender gap and the girls have caught up” is a weird way of saying that girls and boys have never had a meaningful gender gap for as long as the test has been used.
5) Reeves says that boys are doing better at the SATs, “But this gap has narrowed sharply, down to a thirteen-point difference in the SAT” (page 20). Reeves then cites the 2021 Suite of Assessments, which confirms the 13-point difference, but does not confirm that it has narrowed sharply. Reeves does not say what the gap used to be. I searched briefly to see if could verify that it has narrowed sharply. All I found was that the gap on the math section has remained virtually unchanged since 1967.
My Predictive Model
I’m predicting college enrollment by the number who are academically capable times the percent who are financially capable times the percent who want to enroll:
academically capable * financially capable * college aspiration
Something of historic magnitude is clearly happening with college enrollment. But changes in learning gaps are not happening at all. So often in data analysis there’s the challenge of disentangling correlation versus causation. But in this case, there is neither causation nor correlation.
I’m curious how this conversation will play out. Colleges are already going bankrupt. Applications are down overall. In 2026 - 2027 we’re going to see a decline in applications due to the decline in births during the 2008 Financial Crisis. College administrators are in a tough position. Universities are financially two strokes from midnight and soon they’ll have to decide between confronting a highly contentious topic (that men have been an underrepresented population on college campuses for three generations) or risk losing their jobs because their institution has gone bankrupt by hemorrhaging male customers.
If you have suggestions on how to predict the enrollment rates, please let me know.
It's a bit different here in Australia.
I only have (very!) incomplete data but it shows (suggests?) a big fast shift from approximate equality to a large difference in results between girls & boys. I have a graph for one state & I have seen data for another but lost it. In both cases the shift coincided with moving from exams set & marked externally to marks being given by one's teacher. However this is ancient history now - 1970s in one case & 1990s in another.
I understand something similar occured in the UK: http://empathygap.uk/?p=3810
In more recent times (since 2008) the proportion of boys failing to achieve minimum standards has continued to get worse compared to girls. (Australia's education system is in a bit of a mess. Standards for both boys & girls decline but more so for boys.)
Similarly entry to Tertiary education.
The number of boys being punished, suspended and expelled are also grounds for concern here.
A) I feel like I'm coming in half way through a movie, this is the first article of yours I've read :)
B) I somewhat understand the purpose of comparing men and women using the standard deviation, and I like the illustrative use of the blue/green group and asking which group is 0.8 inches taller, but I'm still not sure why it *doesn't* matter that women are 0.8 Anecdotal Inches taller.
C) Is it possible that women are very lightly (1/3 Standard Deviation) ahead of men in important aspects of "being good at school" and that this has a large effect on the college aspirations in your algorithm? If that's the case, in my mind it discounts your argument that boys and girls are neck-and-neck by some percentage. I think it matters if there is a 1% difference at T=0 and a 30% difference at T=10. The importance of T=1 to 9.
C) You are acknowledging a higher graduation rate in women (70:100, men-to-women) and I think that tells a significant, explanation-generating story for why society is weirdly skewed. To me, this is the 30% effect at T=10
D) I think that things which we do/should care about are invisible to these chosen metrics. For example, the broke English major trope or the gender studies/masters of education working at Starbucks kind of highlights that graduation rates don't really mean anything to prosperity. I know a decent number of people with a lot of useless masters and PhDs working $50-60k/year jobs, and most of the people I know making >$100k don't have masters degrees (My group is probably a statistical anomaly).